Segmentation via Thresholding
- Gray level thresholding is the simplest segmentation process.
- Many objects or image regions are characterized by constant reflectivity
or light absorption of their surface.
- Thresholding is computationally inexpensive and fast.
- Thresholding can easily be done in real time using specialized hardware.
- Complete segmentation can result from thresholding in simple scenes.


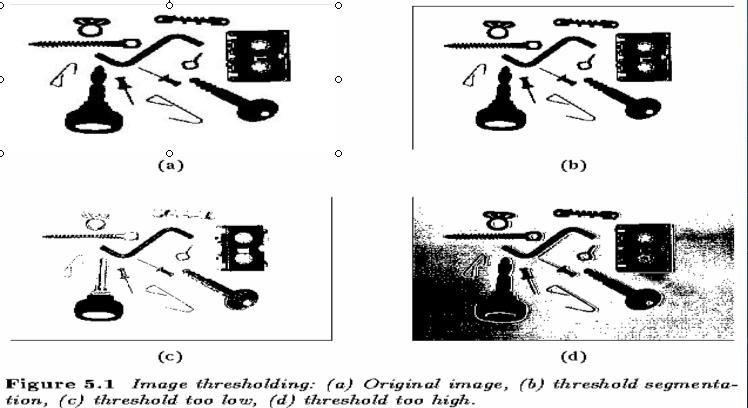
Algorithm
for(each pixel f(i,j) of image)
if f(i,j) >= T
is an object pixel
else
is a background pixel
|
- Correct threshold selection is crucial for successful threshold
segmentation
- Threshold selection can be interactive or can be the result of some
threshold detection method
Single global threshold
- Successful only under very unusual circumstances
- gray level variations are likely - due to non-uniform lighting,
non-uniform input device parameters or a number of other factors.

Variable thresholding
- (also adaptive thresholding), in which the threshold value varies
over the image as a function of local image characteristics, can produce the
solution in these cases.
- image f is divided into subimages fc
- a threshold is determined independently in each subimage
- if a threshold cannot be determined in some subimage, it can be
interpolated from thresholds determined in neighboring subimages.
- each subimage is then processed with respect to its local threshold.

Band-thresholding
- segment an image into regions of pixels with gray levels from a set D and
into background otherwise

- Can also serve as border detection
Multithresholding
- resulting image is no longer binary
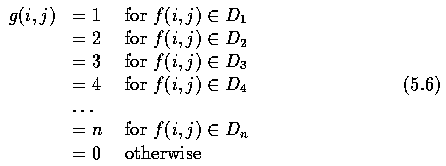
Semithresholding
- aims to mask out the image background leaving gray level information
present in the objects

Threshold detection methods
- If some property of an image after segmentation is known a priori, the
task of threshold selection is simplified, since the threshold is chosen to
ensure this property is satisfied.
- Example A printed text sheet where we know that characters of the
text cover 1/p of the sheet area.
P-tile-thresholding
- choose a threshold T (based on the image histogram) such that 1/p of the
image area has gray values less than T and the rest has gray values larger
than T
- in text segmentation, prior information about the ratio between the sheet
area and character area can be used
- if such a priori information is not available - another property, for
example the average width of lines in drawings, etc. can be used - the
threshold can be determined to provide the required line width in the
segmented image
More complex methods of threshold detection
- based on histogram shape analysis
- bimodal histogram - if objects have approximately the same gray
level that differs from the gray level of the background
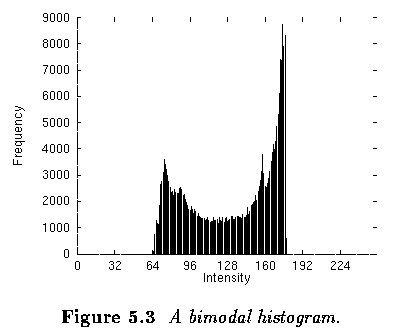
- Bimodality of histograms
- to decide if a histogram is bimodal or multimodal may not be so simple in
reality
- it is often impossible to interpret the significance of local histogram
maxima
- Bimodal histogram threshold detection algorithms
- Mode method - find the highest local maxima first and detect the
threshold as a minimum between them
- to avoid detection of two local maxima belonging to the same global
maximum, a minimum distance in gray levels between these maxima is usually
required
- or techniques to smooth histograms are applied
- Histogram bimodality itself does not guarantee correct threshold
segmentation
Optimal thresholding
- based on approximation of the histogram of an image using a weighted sum
of two or more probability densities with normal distribution
- The threshold is set as the closest gray level corresponding to the
minimum probability between the maxima of two or more normal distributions,
which results in minimum error segmentation
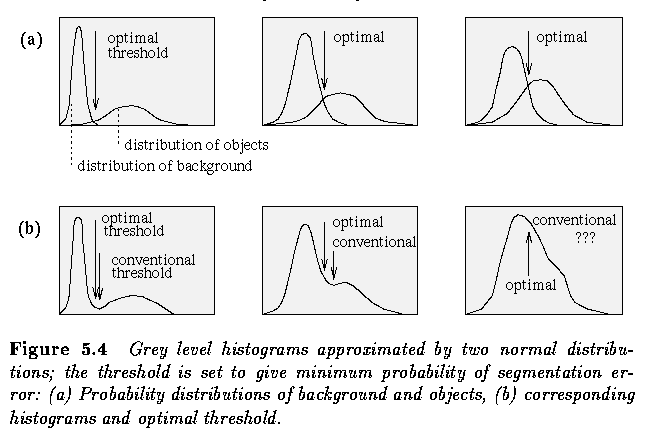
- Problems - estimating normal distribution parameters together with
the uncertainty that the distribution may be considered normal.
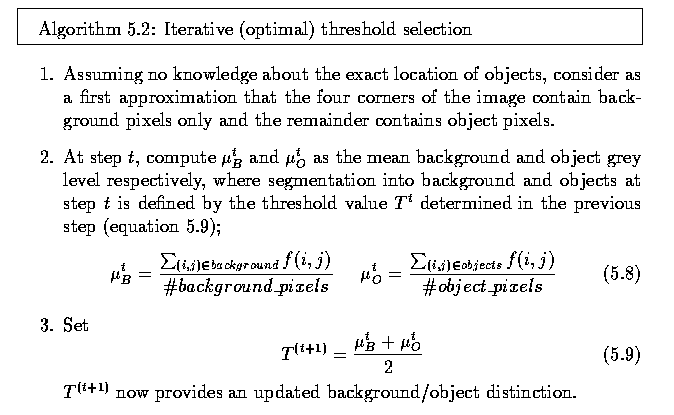

- The method performs well under a large variety of image contrast
conditions
Example - Brain MR image segmentation
- A combination of optimal and adaptive thresholding
- determines optimal gray level segmentation parameters in local subregions
for which local histograms are constructed
- gray-level distributions corresponding to n individual (possibly
non-contiguous) regions are fitted to each local histogram that is modeled as
a sum of n Gaussian distributions so that the difference between the modeled
and the actual histograms is minimized

Variable g represents gray level values from the set G of images gray
levels, ai, &sigmai and ยตi denote
parameters of the Gaussian distribution for the region i.
The optimal parameters of the Gaussian distributions are determined by
minimizing the fit function F

Applied to segmentation of MR brain images, three segmentation classes - WM,
GM, CSF
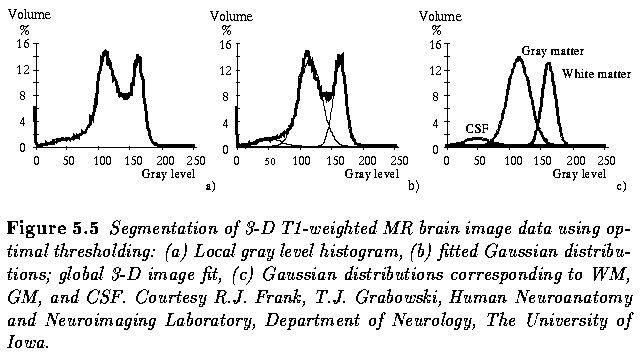
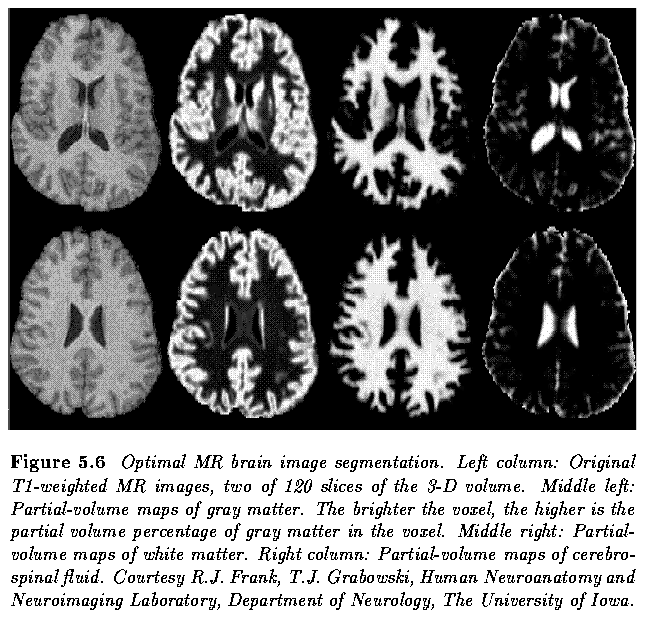
Multispectral thresholding
- Multispectral or color images
- One segmentation approach determines thresholds independently in each
spectral band and combines them into a single segmented image.
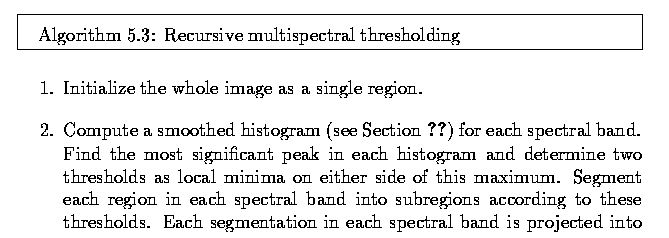
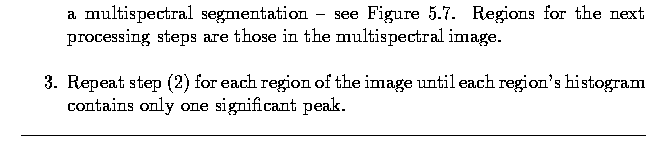
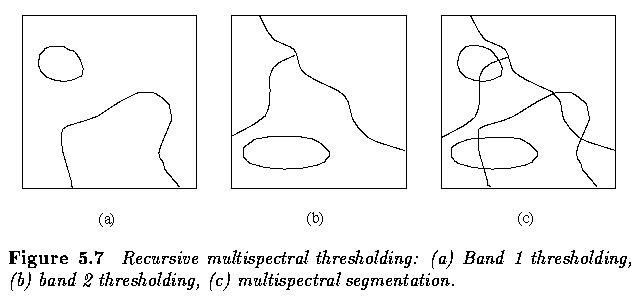
Hierarchical Thresholding
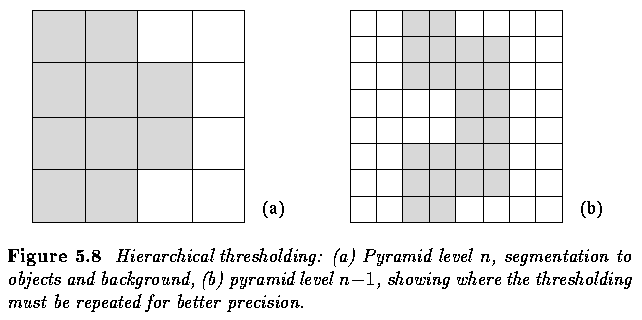

|