Example to Understand CNN - determine if image has X or O
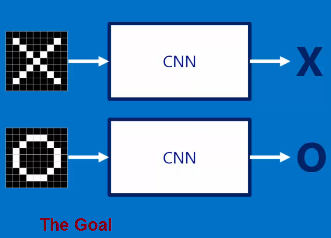
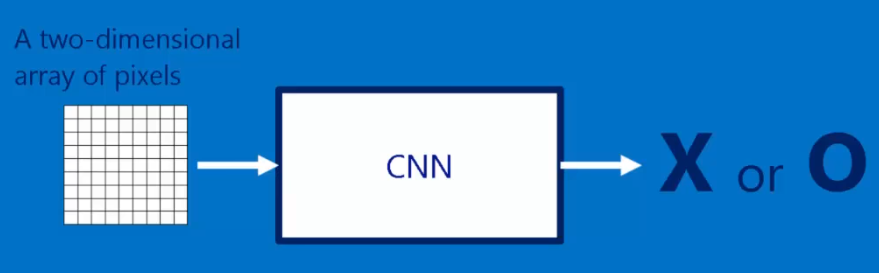
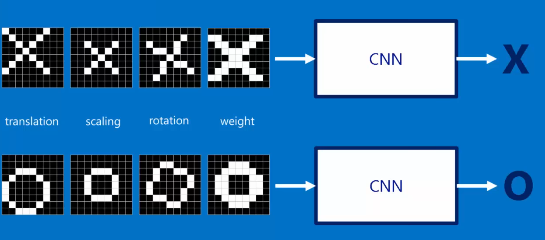
How much training data --- examples for some forms of object recognition - could go into 100,000 samples or more. Sometimes like 10,000 or maybe for the really really simple example we show here we might get away with 200?
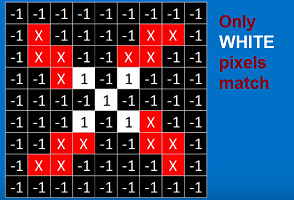
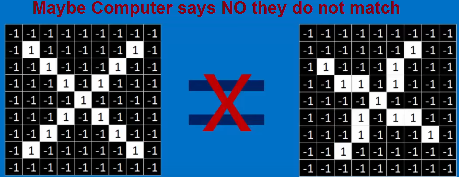
CNN --- in "some way" can be thought of breaking it down and matching parts of image to each other ---
-
The matching is done through convolving the image with the MxM mask/filter
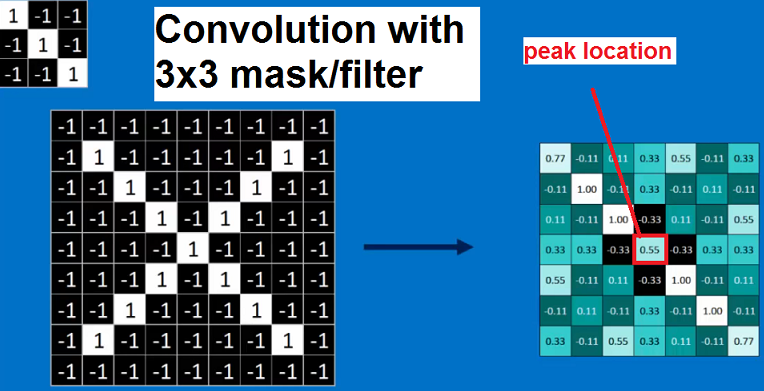
QUESTION: How many Filters do we apply at each level?????? ---here we show 3 filters/masks
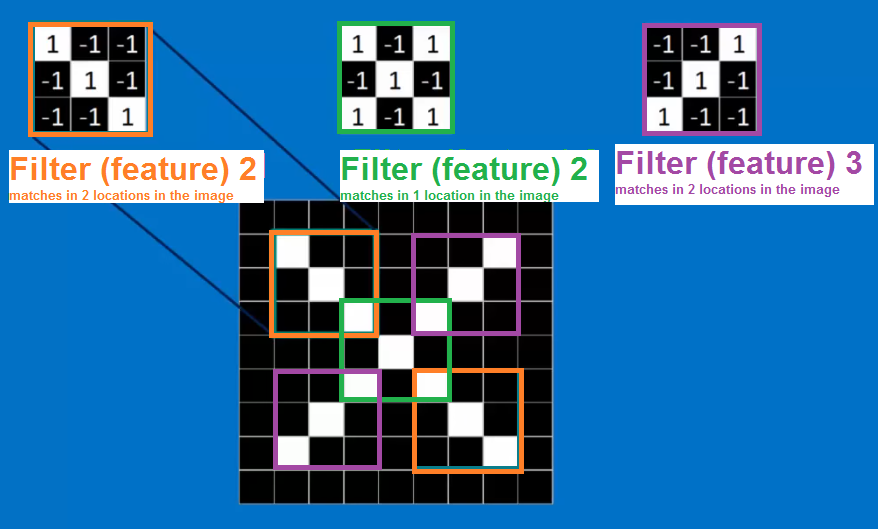
RESULTS
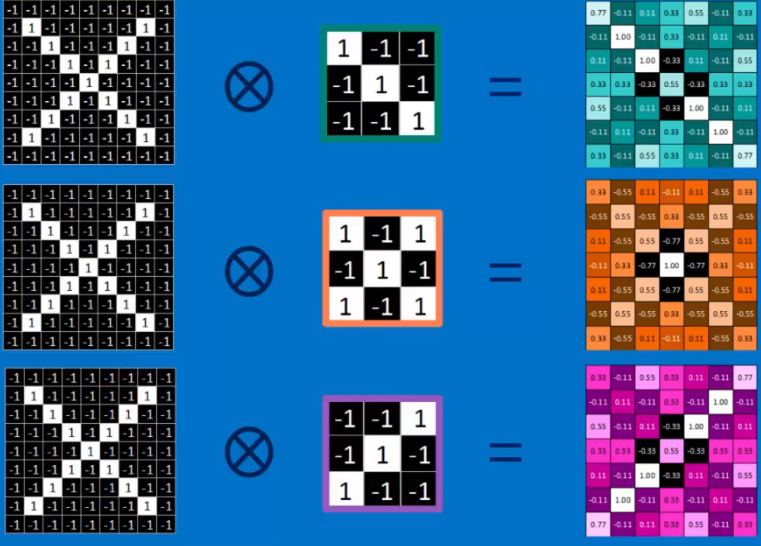
These MxM filters/masks are applied convolutionally at each layer in our CNN - in the case of our input image we apply this at each raw input pixel (at each red, green, blue if color) and we can apply only 1 MxM filter or could have multiple filters like shown above the case is 3 -- in our binary image input this would result in 3 times the amount of input data for the 3 images.......
<< example where input image 32x32 pixels and have THREE MxM filters convolve with yielding 6 times data. Note here MxM =5x5 and we can not convolve with the first 2 and last 2 rows and columns (boundaries of image) --> so get THREE 28x28 size data values ---- YIKES bigger --- I want the output 2 have 2 nodes "X" and "Y". What am I going to do??? --> CNN does downsampling at each layer called Max Pooling
MAX Pooling - typically do on 2x2 (or maybe 3x3)
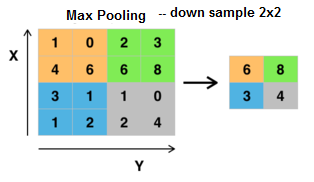
NOW we have THREE 14x14 data sets --thats a bit better
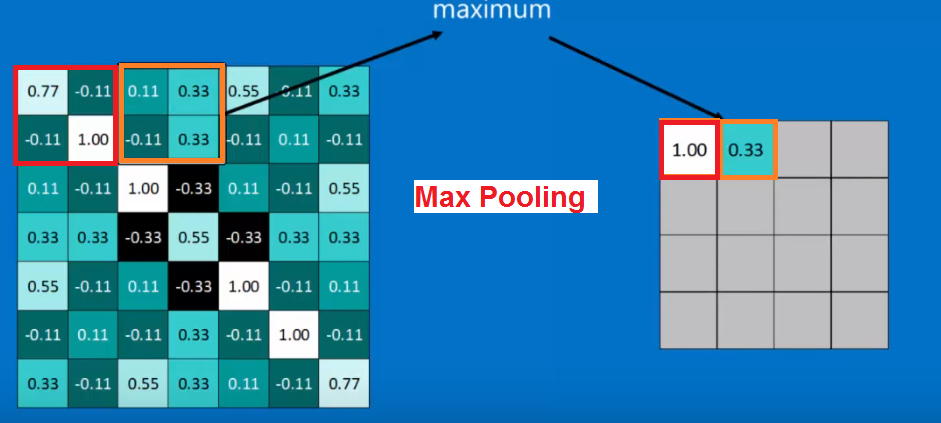
RESULTS
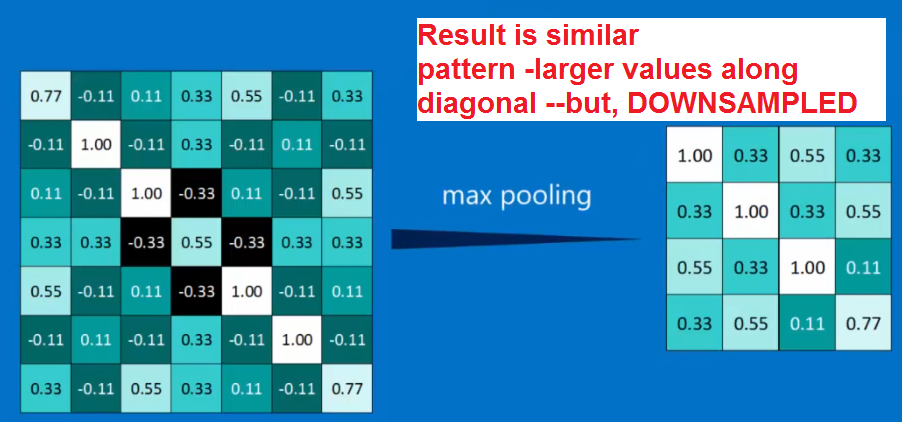
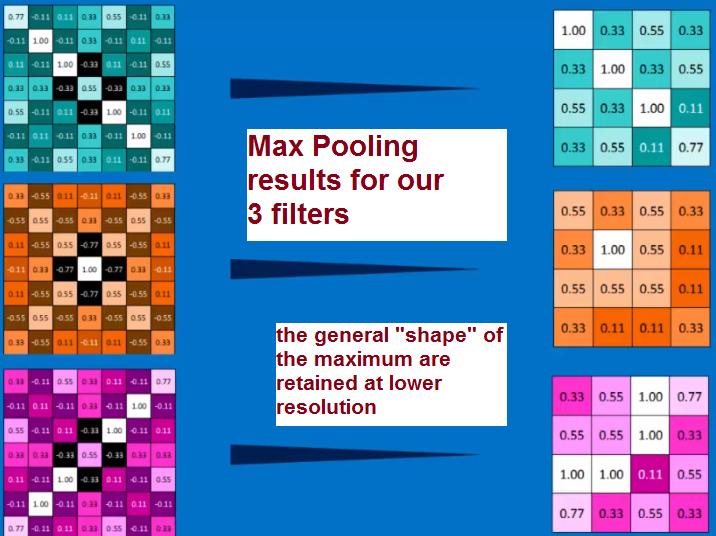
WAIT we not done --- we must introduce a non-linearity and do so for CNN at each data sample by applying Rectified Linear Units: set values < 0 to 0
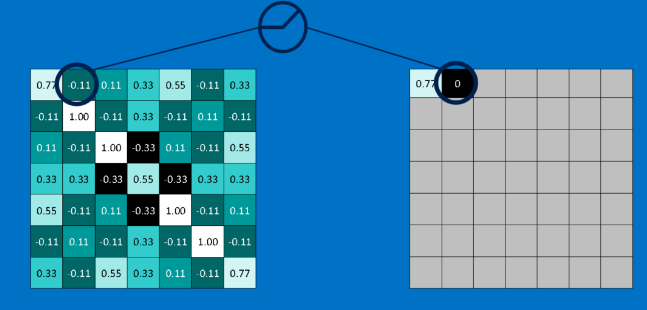 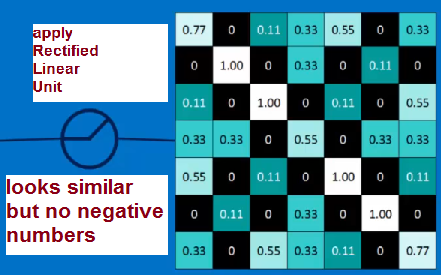
Convolution with set of (1-*) MxM filters + nonlinear + max pooling (downsample)
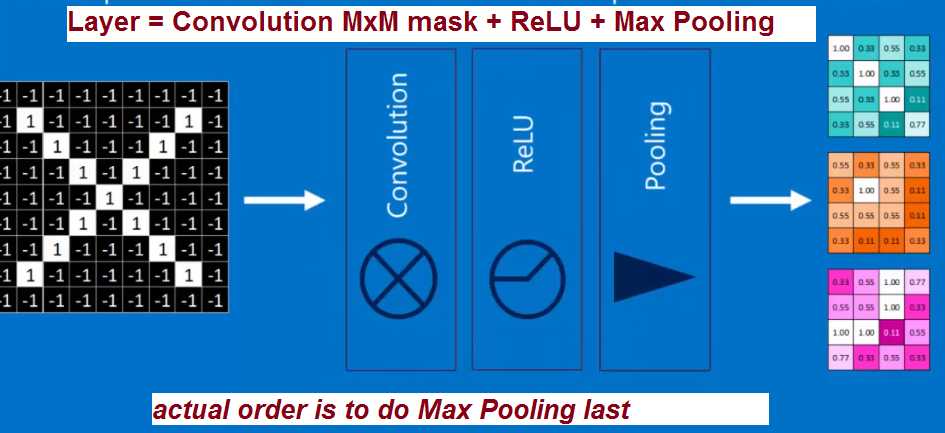
Example with 3 Layers using convolution masks
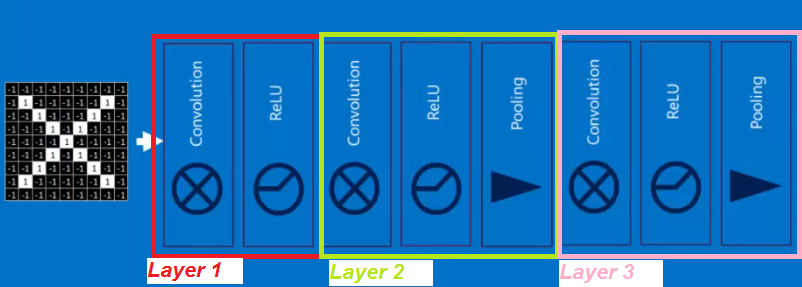
(you can have more than one layer at the end that is fully connected like this --but, minimum is 1)
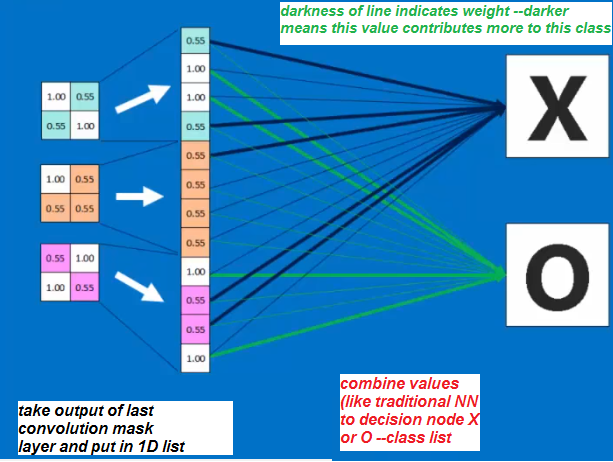
After Training --making the decision at last layer
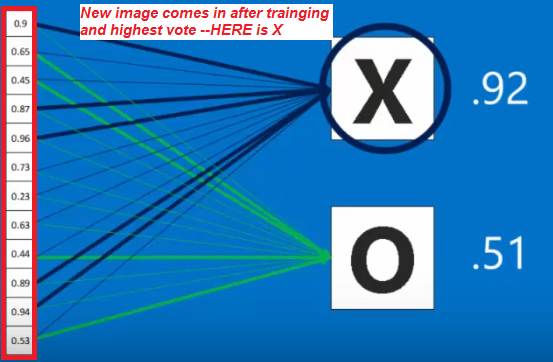
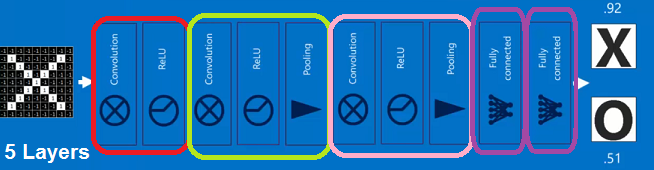
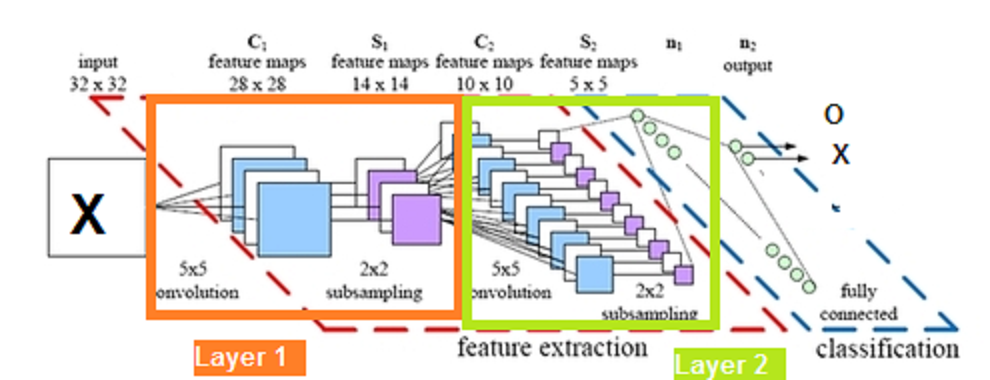
-
Question 1: How many layers of each type (convolution and fully connected)?
hard to answer - minimum 3 largest maybe 6-15 (but, that is changing) . For sure the more layers, the longer to train and in general more data needed. More complex the problem more layers. Simpler the problem less layers needed. Minimum 1 fully connected layer. NOT LEARNED, you decide
-
Question 2: How many masks at each layer?
hard to answer - could learn this. Minimum obviously 1. NOT LEARNED, you decide
-
Question 3: what size is the mask?
hard to answer - minimum is 3x3. Could be larger but, should not exceed 25% of image (?? of thumb). larger mask means larger scale pattern looking to match via convolution. NOT LEARNED, you decide
-
Question 4: What is content of MxM mask?
you learn this through training (backpropogation like traditional NN, use error between output and desired output to alter the parameters) LEARNED
-
Question 5: What are the weights in the fully connected layers?
you learn this through training (backpropogation like traditional NN, use error between output and desired output to alter the parameters) LEARNED
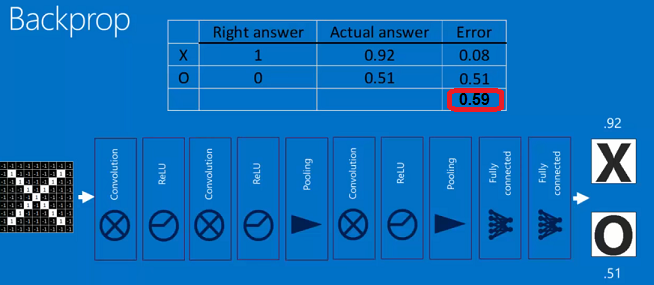
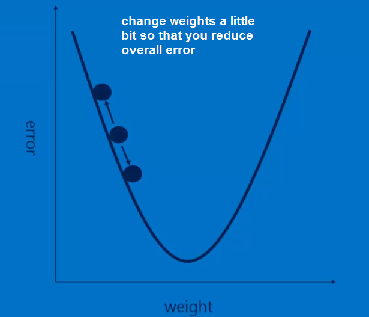
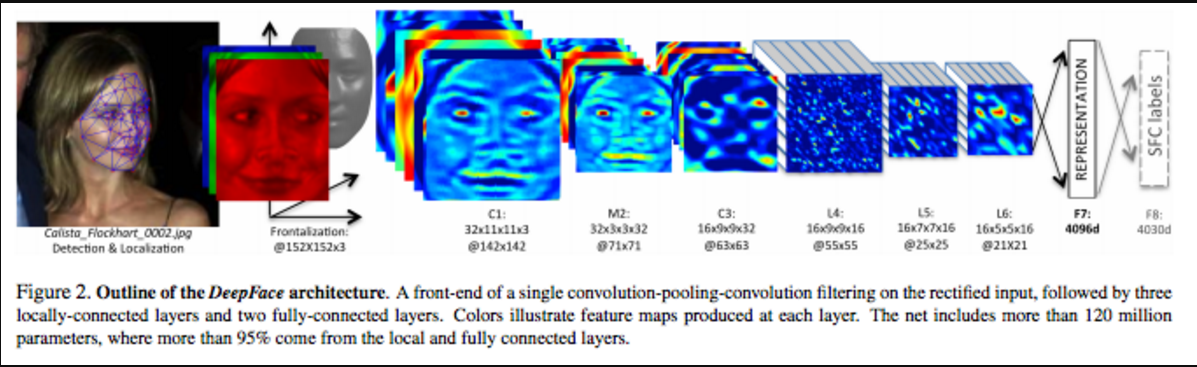
OUTCOME: CNN capture "local" spatial patterns at different scales (downsampling-max pooling) --- good for Image Applications
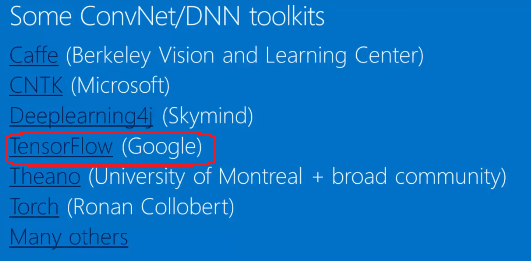
|